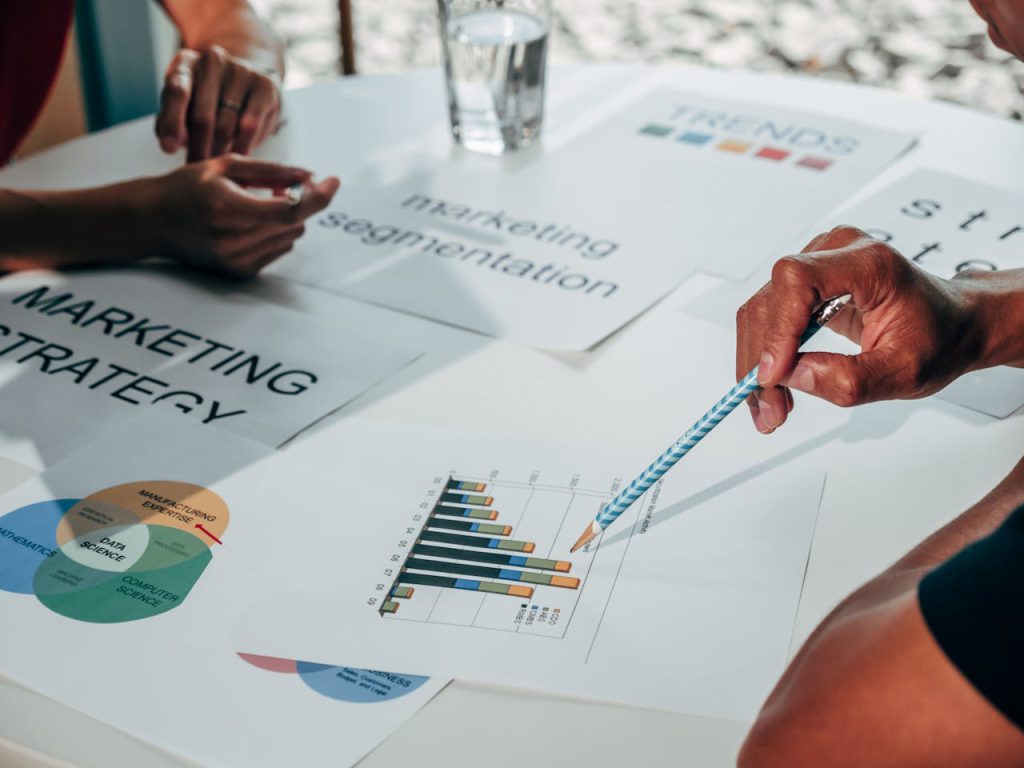
Data quality assessments:Ensuring data quality is essential for meeting user requirements and achieving the intended purpose of data collection and analysis. High-quality data are reliable, valid, precise, timely, and maintain confidentiality, forming the foundation for effective decision-making and program success. A Data Quality Assessment (DQA) is a systematic process used to evaluate the quality of data against these dimensions. By identifying strengths and weaknesses in data management systems, a DQA helps project partners enhance data reliability and integrity while ensuring the data are suitable for monitoring and evaluation purposes. This guide provides insights into the importance of data quality and the role of DQAs in improving data systems and decision-making.
Data quality refers to the degree to which data satisfy user requirements or are suitable for a specific process or purpose.
Quality data are data that are reliable, accurate, precise and complete, provided in a timely manner, valid, and maintain client confidentiality.
Defining DQA in data quality assessments.
DQA is a periodic review that helps project partners determine and document “how good the data is” and also provides an opportunity for capacity-building of implementing partners.
USAID definition in data quality assessments.
A DQA is a process to help project partners understand the strengths and weaknesses of their data and the extent to which the data can be trusted to influence management decisions.
It refers to the standard practice for assessing data quality, documenting any limitations in data quality, and establishing a plan for addressing those limitations.
This is a strategy that is used by project partners to assess the strengths and weaknesses of the data in relation to data quality dimensions (validity, integrity, precision, reliability, timeliness).
Purpose of DQA
It is important to conduct DQA on a regular basis at all stages of project cycle for purposes of;
Verifying the quality of reported data for key indicators.
Assessing the ability of data management systems to collect, manage and report quality data.
Putting up corrective measures for strengthening the data management and reporting system and improving data quality.
Capacity improvements and performance of the data management and reporting system to produce quality data.
Indicators
Indicators can be measured or expressed in form of
- Number
- Ratio
- Percentage
- Average
- Rate
- Index
Data Quality Dimensions.
A DQA is conducted to understand and document the extent that data meet the five data quality standards/dimensions:
Validity: Data should represent the intended result clearly and adequately.
Validity: Representing the Intended Results Clearly and Adequately.
Validity refers to the extent to which data accurately represent the intended results or objectives of a measurement. Data are considered valid when they clearly, adequately, and truthfully reflect the phenomenon they are intended to measure. For data to be valid, the processes, tools, and methodologies used in their collection must be designed to capture the true value of the intended outcome with minimal distortion.
Ensuring validity requires careful attention to definitions, measurement tools, and data sources. Proxy indicators may be used when direct measurement is challenging, but their relevance and accuracy must be evaluated to ensure they effectively reflect the intended result. For instance, using “condoms distributed” as a proxy for “condom use” in an HIV prevention program requires understanding its limitations in fully capturing the behavior it aims to measure.
Key Considerations for Validity:
- Data collection tools must align with the objectives of the measurement.
- Definitions of terms and indicators must be precise and consistently understood by all stakeholders.
- Data must be free from bias introduced during collection, transcription, or analysis.
Validity ensures that data serve their purpose, accurately reflect the realities they are meant to represent, and provide a strong foundation for decision-making and evaluation.
Integrity:
Data should have safeguards to minimize risk of bias, transcription error, or data manipulation.
Integrity: Safeguarding Data Against Bias, Errors, and Manipulation
Integrity refers to the trustworthiness and authenticity of data, ensuring they are accurate and free from intentional or unintentional distortion. For data to have integrity, robust safeguards must be in place to minimize the risk of bias, transcription errors, or manipulation at every stage of the data lifecycle—collection, storage, analysis, and reporting.
Maintaining data integrity requires transparent processes, ethical practices, and secure systems that protect against both human and technological vulnerabilities. Errors or biases introduced during data handling, whether accidental or deliberate, can compromise the reliability of results and lead to flawed decision-making.
Key Measures to Ensure Integrity:
- Establishing clear data governance policies and ethical standards.
- Implementing quality control checks during data collection, entry, and analysis.
- Ensuring secure storage and access protocols to prevent unauthorized alterations.
- Training personnel to follow standardized procedures and uphold ethical guidelines.
By safeguarding against potential threats such as time pressures, incentives to manipulate data, or technical failures, organizations can preserve the integrity of their data, ensuring that it reliably reflects the true conditions it is intended to measure.
Precision:
Data should have a sufficient level of detail to permit informed management decision making.
Precision: Ensuring Sufficient Detail for Informed Decision-Making
Precision refers to the level of detail and exactness in data, ensuring it is adequate to support effective and informed management decisions. Precise data provide clear and specific insights that allow stakeholders to assess trends, measure progress, and make decisions with confidence.
For data to be precise, the margin of error must fall within an acceptable range that aligns with the goals of the program or analysis. The level of detail in the data must also match the needs of the intended purpose, whether it involves disaggregating data by demographics or tracking changes over time.
Key Considerations for Precision:
- Data collection methods should be designed to minimize errors and provide granular information.
- The acceptable margin of error should be clearly defined and reported, particularly when measuring small changes.
- Precision should be balanced with feasibility to ensure the data are detailed enough without being overly burdensome to collect.
Precision ensures that data are not only accurate but also specific enough to guide meaningful action and provide clarity for decision-makers at every level.
Reliability:
Data should reflect stable and consistent data collection processes and analysis methods over time.
Reliability: Ensuring Stability and Consistency Over Time
Reliability refers to the consistency and dependability of data, ensuring that the same results are achieved under similar conditions using stable data collection processes and analysis methods. Reliable data reflect a system in which variations are minimized, allowing decision-makers to trust the results and draw accurate conclusions.
For data to be reliable, the processes and tools used must remain consistent across different times, locations, and personnel. Any deviations in methodology, training, or interpretation can introduce inconsistencies that compromise the reliability of the data.
Key Considerations for Reliability:
- Standardization: Use uniform tools, protocols, and procedures for data collection and analysis.
- Training: Ensure that all personnel involved in data collection and management are well-trained and understand the purpose and methods.
- Quality Control: Implement regular checks to identify and correct inconsistencies in data collection or handling.
- Documentation: Maintain detailed records of processes to ensure they can be replicated accurately.
Reliable data enable program managers and stakeholders to confidently monitor progress, evaluate outcomes, and compare results over time, ensuring that decisions are based on a stable foundation of consistent information.
Timeliness:
Data should be available at a useful frequency, should be current, and should be timely enough to influence management decision making.
Timeliness: Providing Current Data to Support Decision-Making
Timeliness refers to the availability of data at the right time, ensuring it is delivered with sufficient frequency and currency to support informed management decision-making. Timely data is critical for assessing program performance, identifying issues, and implementing corrective actions before opportunities for impact are lost.
For data to be timely, it must be collected, processed, and reported in a manner that aligns with the needs of stakeholders and the pace of program activities. Delays in data availability can render it obsolete, limiting its value in guiding decisions.
Key Considerations for Timeliness:
- Frequency: Data should be collected and reported at intervals that match the decision-making cycle.
- Relevance: Data must be up-to-date and reflect the most recent program activities and outcomes.
- Efficiency: Streamline data collection and processing systems to reduce delays without compromising quality.
Common Challenges to Timeliness:
- Slow data entry or report generation processes.
- Limited resources for timely data collection and analysis.
- Outdated information systems that hinder efficient reporting.
Timeliness ensures that data remains actionable, allowing organizations to respond promptly to emerging trends, evaluate ongoing efforts, and make well-informed decisions that maximize program effectiveness.
Validity (Results satisfy objectives)
Validity means that whatever is being measured, is actually what we have intended to capture or measure i.e., the results must satisfy and be in accordance with the objectives of the test.
Data should clearly and adequately represent the intended results.
Validity/accuracy of data is the degree to which data correctly reflects the true value or how close the data is to the true measurement.
While proxy data/indicators may be used, one must consider how well the data measure the intended result.
The key question on validity is whether the data actually represent what they are supposed to represent.
Examples of Data Validity: The age of the beneficiary in the database is the true age of the person.
For data to have measurement validity, data measurement tools and procedures must have been well designed and must limit the potential for errors.
You could have data validity issues if you answer “Yes” to any of these questions:
Did respondents have trouble understanding the instructions on how to fill the forms/registers?
Are data incomplete?
Were data altered in transcription?
Threats or barriers to Validity
1) Definitional issues
Remember, any word in an indicator can be understood differently (trained, service, people, number of).
If these words in the indicator definition are not defined completely enough and also communicated across all users of this data, there can be definitional issues.
2) Proxy measures
Some results, especially outcome level results cannot be captured by a simple number or count. It is a measure of change and this can be difficult
Example: HIV prevention intervention can be measuring ‘condom use’
For some obvious reasons, we cannot measure actual condom usage;
We therefore find the closest measure to that, our proxy measure.
In this example we sometimes use ‘condoms distributed or sold’ as our proxy measure.
3) The data source is where the data are borne
When various data sources are involved in one aggregated measure, what is actually being measured can slightly vary from place to place. This can influence the validity.
Reliability
What is meant when we say something is reliable?
What makes a person reliable?
Reliability (Results are consistent)
Reliable measures are measures that are concerned with consistency: doing things or behaving the same way over a period of time.
In data quality, we ask ourselves, “Are we measuring the same thing in the same way each time?”
Data should reflect stable and consistent data collection processes and analysis methods over time.
For a data set to be reliable, data collection processes must be stable and consistent over time, with reliable internal quality controls in place and data procedures handled in a transparent manner.
The key issue is whether M&E team and Program Managers would come to the same conclusions if the data collection and analysis process were followed accordingly.
People, Places, and Time affect reliability of data
Collection methodologies, when not standardized or understood in the same way across several collection areas, can affect data reliability.
People use different collection methods. People use the same collection methods, differently. Collection methodologies may also need to account for data collection sites that are in different places: geographically, urban, rural?
Training Package- threats to reliability of the data
You can have all the data quality assurance measures in place, but if personnel around data collection are not fully trained, there can still be a wide range of threats to the reliability of your data.
More importantly, they must understand the basis behind why they are collecting what they are collecting.
Data Triangulation- threats to reliability of the data
Once we have the data on hand, most often we will need to do something with this data to turn it into usable and valuable information.
We put it into context, we aggregate it with the same data type from other sources, or we triangulate with other data to track trends over time, etc.
When doing this, if we do not do it consistently, we introduce threats to reliability.